In the Laboratory of Analysis of Systems for the Assessment of Reliability, Risk and Resilience (LASAR3) at Politecnico di Milano we develop methods for risk and resilience assessment, reliability and availability analysis, prognostics and health management and maintenance by leveraging all available knowledge, information and data for the reliability, risk and resilience assessment of components, systems and critical infrastructures.
DISCOVER MORE
New PhD position opening!
The increased availability of data from industrial equipment and the grown ability to treat these data by artificial intelligence (AI) methods have opened the doors for the development and application of predictive maintenance in several industrial sectors, like nuclear, oil and gas, energy, electronics and transportation. Practical implementation of predictive maintenance entails trustworthiness of the AI model outcomes.
The PhD position, available in LASAR3, aims at developing new AI analytics for predictive maintenance based on the powerful emerging techniques of Deep and Transfer Learning, Physics-Informed Neural Networks and Generative Adversarial Networks for improving prediction accuracy, especially in case of imbalanced data, i.e. dew degradation and failure data (challenge i); Deep Ensembles and Bayesian Neural Networks for treating the uncertainty in AI models (challenge ii) and eXplainable Artificial Intelligence
📘 Title of the research: TRUSTWORTHY ARTIFICIAL INTELLIGENCE FOR PREDICTIVE MAINTENANCE OF INDUSTRIAL EQUIPMENT
🔗 Call description: link
🔗 On-line Application: link (online services). After registering, you can use your Person Code and password to access the PhD application: online services – Application forms – Application for “bandi aggiuntivi” PhD programmes
📆 Grant starting date: 1 May 2025
📆 Deadline for application: 18 March 2025 (2pm CET)
Continuing Education Course
Milan, Italy, May 5-9, 2025
Artificial Intelligence for Energy Systems
Join us for the I edition of the Course “Artificial Intelligence for Energy Systems” to learn about Artificial Intelligence (AI) algorithms and tools to extract information useful for operating the energy components and systems in an efficient, reliable, safe, and sustainable way.
The course, directed by Prof. Enrico Zio, is organized by the Department of Energy – Politecnico di Milano, with the support of ARAMIX | AI for Efficiency by Datrix Group, MINES Paris-PSL University, Centre de Recherche sur les Risques et les Crises (CRC) (France), and IEEE Italy Section – Reliability Society.
The goal of the course is to provide scientists, operation/maintenance engineers, asset/facility/energy managers, R&D and sustainability managers, researchers, and PhD students with methodological competencies, analytical skills, and practical knowledge on the existing algorithms and available computational tools of AI for system modeling, analysis, simulation, virtual sensing, component health management, forecasting, control and remote auditing, reliability analysis and risk assessment management, multi-objective and multi-level optimization, and decision support systems.
Registration (within April 16th, 2025):
https://www.corsoaienergysystems.energia.polimi.it/registration-and-costs/
The European Safety and Reliability (ESREL) and Society for Risk Europe (SRA-E) Conference
Stavanger, Norway, June 15-19, 2025
This year’s theme is “Advancing risk, safety and reliability sciences, with applications – from component, to system, to society”. The LASAR3 Group is organizing the following special sessions:
Reliability, Risk and Resilience of Cyber-Physical Systems
Climate Change and Extreme Weather Events: Impacts on Critical Infrastructure Risk and Resilience
Resilience of Cyber-Physical Systems (CPSs) to security attacks
Artificial Intelligence, Meta-Modelling, and Advanced Simulation for the Safety Analysis of Nuclear Systems
Bayesian Networks Modelling for Reliability and Risk Assessment
Domain Adaptation Methods for Prognostics and Health Management (PHM) and Predictive Maintenance
Physics-Informed Machine Learning for RAMS
Natural Language Processing for RAMS applications
Reinforcement learning for RAMS
Explainable Artificial Intelligence (XAI) for Reliability, Availability, Maintainability and Safety (RAM) Applications
Don’t lose the opportunity to participate!
Click here for more information
SUBMISSION DEADLINE: October 15th, 2024
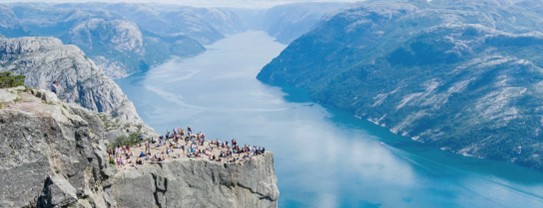
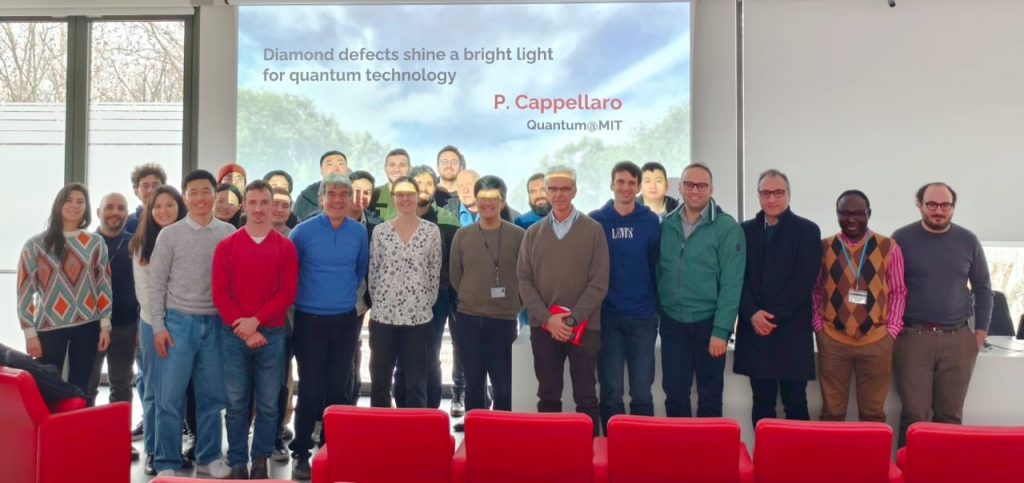
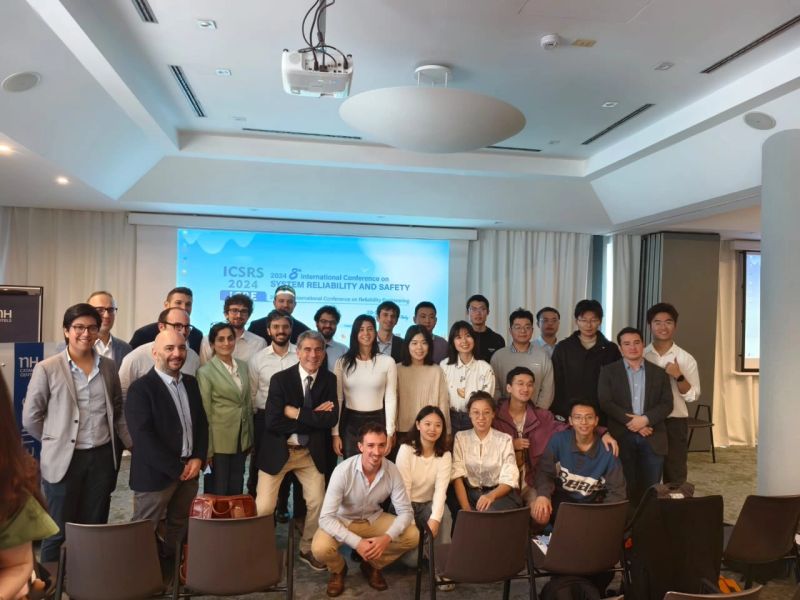
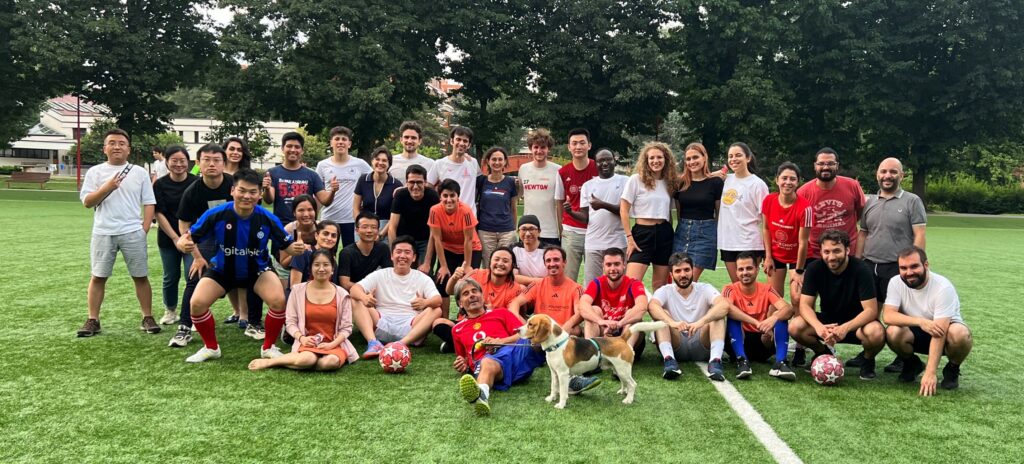
Follow us on LinkedIn